" />
class: center, middle, inverse, title-slide .title[ # 데이터과학자가 갖춰야할 기술 ] .author[ ### 김진섭;
<i class="fab fa-github faa-float animated "></i>jinseob2kim
] .institute[ ### 차라투(주) ] --- layout: true <div class="my-footer"><span><a href="https://www.zarathu.com">Zarathu Co.,Ltd</a>                             <a href="https://github.com/jinseob2kim">김진섭</a></span></div> --- # Executive summary .large[ 분석: **의학 + 연구는 R**추천 - 데이터 전처리는 **[data.table](https://blog.zarathu.com/posts/2022-02-11-datatable/)** 과 `%>%`. - R로 ppt/xlsx 만들기(분석결과 반출) - 일반, 반복측정, 표본추출, Propensity, meta, 시계열, ML - 코드관리는 Github 서비스: 리포트(Rmarkdown), 웹 애플리케이션(Shiny) 서버: 분석환경 or 웹 서비스 - 리눅스, 클라우드, Docker ] --- # 소개 .large[ 의학연구지원법인 차라투 대표 (5년차) ] <center> <img src="self.png" width=100%></a> </center> --- # 맞춤형 통계웹  --- # [공개 통계웹](https://openstat.ai) 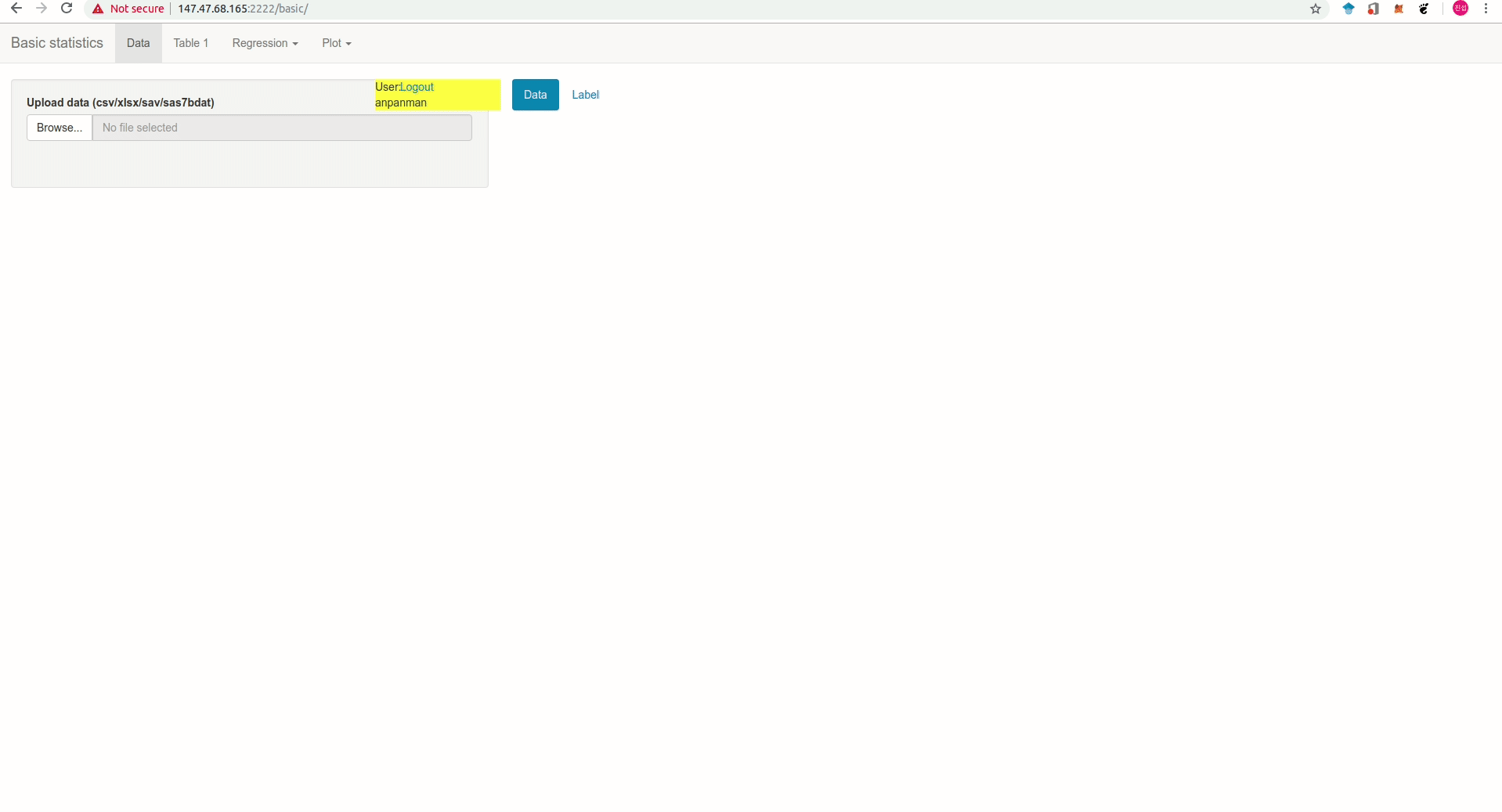 --- # 주요 계약 병원 - 강동성심병원 원내통계 전담 - 길병원 원내통계 10개 전담 - 서울대병원 순환기내과, 삼성서울병원 정신건가의학과 통계자문계약 - 국립중앙의료원 암통계 대시보드 개발 제약회사 - 신라젠, 오츠카제약 --- # 주요 논문 성과 .large[ SCI 논문 200건 지원 ] <center> <img src="https://jinseob2kim.github.io/lecture-general/statreview/paper.png" width=80%></a> </center> --- # NEJM .large[ 심혈관중재시술 전략비교 ] >삼성서울병원 이주명, 최기홍 교수님을 도와 두 심혈관중재시술(PCI) 전략을 비교, 다기관 고려한 stratified cox 와 competing risk analysis 를 수행하고 관련 통계자문을 제공하였습니다. 본 연구는 New England Journal of Medicine(NEJM, IF 176.079) 에 게재되었습니다. <center> <img src="https://oopy.lazyrockets.com/api/v2/notion/image?src=https%3A%2F%2Fs3-us-west-2.amazonaws.com%2Fsecure.notion-static.com%2Fbf058199-3a3a-49ab-990b-66dc135fb739%2FUntitled.png&blockId=5f2b13d8-6953-411a-a245-73e0e8d1afc7" width=80%></a> </center> --- # Lancet .large[ Statin 병용요법 비열등성검정 ] > 연세의료원 심장내과 홍명기교수 팀을 도와 high-intensity statin 단일요법과 ezetimibe + moderate-intensity statin 병용요법을 비교, (1)비열등성검정 샘플수 계산 및 통계검토와 (2)리비전 통계분석을 지원하였습니다. 본 연구는 The Lancet(IF 202.731) 에 게재되었습니다 <center> <img src="http://k.kakaocdn.net/dn/boaUap/btrHDQhI2j1/zVeZ4Mv3DkybiLejdtJ5D1/img_xl.jpg" width=80%></a> </center> --- # JAMA .large[ Statin 치료전략 비교 ] > 연세의료원 심장내과 홍명기교수 연구팀을 도와 LODESTAR (Low-Density Lipoprotein Cholesterol-Targeting Statin Therapy Versus Intensity-Based Statin Therapy in Patients With Coronary Artery Disease) Trial 을 분석, statin 치료전략을 비교하고 리비전에 필요한 통계자문을 제공했습니다. 본 연구는 JAMA(IF 157.3) 에 게재되었습니다. <center> <img src="https://oopy.lazyrockets.com/api/v2/notion/image?src=https%3A%2F%2Fs3-us-west-2.amazonaws.com%2Fsecure.notion-static.com%2F6b9b7e21-2337-46d8-ab8f-f13d47133125%2FUntitled.png&blockId=02589dc1-49a3-417d-b080-08641f27ce83" width=80%></a> </center> --- # 22년 IITP 연구개발과제 선정 .large[ 2년 10.5억 with 앤틀러, 파이디지털헬스케어 ] <center> <img src="http://k.kakaocdn.net/dn/bsgueW/btrzkqLQ3H2/qQBLFHw769HWkRJyeRWRG1/img_l.jpg" width=70%></a> </center> --- # R&D: statgarten .large[ 총괄책임자 - with 파이디지털헬스케어, 앤틀러 ] <center> <img src="https://jinseob2kim.github.io/lecture-general/APPFGH2022/statgarten.png " width=80%></a> </center> --- # 22년 창업도약패키지 선정 .large[ 1억 ] <center> <img src="http://k.kakaocdn.net/dn/cQa4s2/btrESX34HXo/GJZOCzNJwzo4B4czmb2tKk/img_xl.jpg" width=50%></a> </center> --- # 심평원 공통데이터모델 변환 용역 <center> <img src="http://k.kakaocdn.net/dn/0lm3Q/btrL5xcRF86/3NMzYiiKRwq14HUhDKqXG0/img_l.jpg" width=80%></a> </center> --- # 23년 창업성장 디딤돌, 공개SW 기술확산 지원사업 .large[ 사업화분야, 지원금 2억원 ] - 본 과제를 통해 의학연구와 신약임상시험 특화 분석소프트웨어를 오픈소스로 개발, 활발한 의학연구를 이끌고 바이오헬스산업에 기여하겠습니다. 감사합니다. <center> <img src="https://media.licdn.com/dms/image/D4D22AQGMPAb3SWLuUg/feedshare-shrink_800/0/1683018503870?e=1686182400&v=beta&t=Hn7zs6L5gd40N_G7uS3s4cUR9sRflEb87AUHXa1IIw4" width=80%></a> </center> --- # CDISC ADaM <center> <img src="그림1.png" width=100%></a> </center> --- class: center, middle # 핵심기술 R --- # 프로그램 개발, 블로그 운영 .large[ [R](https://www.r-project.org/) packages - [jstable](https://github.com/jinseob2kim/jstable): 논문용 테이블 만들기 - [jskm](https://github.com/jinseob2kim/jskm): 생존분석 그림 만들기 - [jsmodule](https://github.com/jinseob2kim/jsmodule): 웹에서 하는 통계분석 ] -- .large[ 데이터 분석용 가상머신([docker](https://www.docker.com/) image) - [rshiny](https://github.com/jinseob2kim/docker-rshiny): [Rstudio](https://www.rstudio.com/)와 [shiny server](https://www.rstudio.com/products/shiny/shiny-server/) 가 설치된 이미지. ] -- .large[ [블로그](https://blog.zarathu.com/), 카카오톡 오픈채팅방- 프로그래밍 갤러리 R 유저 모임 ] --- # 110,000 다운로드 <center> <img src="https://github.com/jinseob2kim/lecture-general/blob/master/docs/medical-scientist/cran20221015.png?raw=true" width=80%></a> </center> --- # 공부모임: Shiny 밋업 .large[ https://github.com/shinykorea/Meetup - 월 1회 공부내용 공유. 30회 진행 - 의료/유전학/축산/반도체/게임/IPTV/회계 등 다양한 분야 사람들이 모임. - **경기도 코로나 대시보드 공동작업** - 21년 공개SW기반 **커뮤니티** 지원사업, **韓中日 공개SW** 국제협력 강화 TASK 선정 ] --- # 경기도 코로나 [병상관리 대시보드](https://github.com/shinykorea/corona-sickbed) 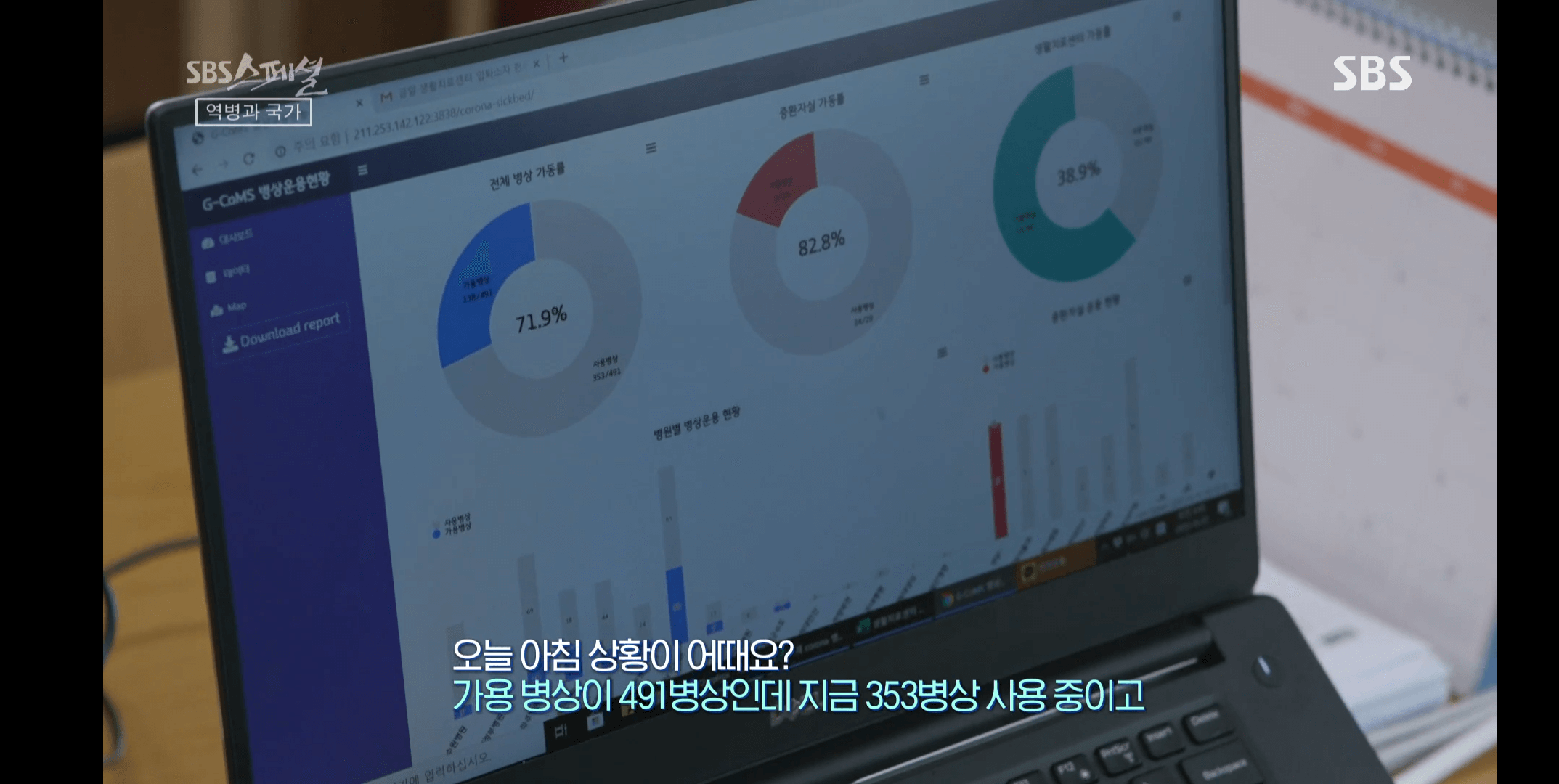 --- class: center, middle # 분석 --- # R vs Python .large[ **의료데이터 + 연구**는 R이 좋다. - 의학분야 최신패키지는 R first - 강력한 전처리 패키지 존재: [tidyverse](https://blog.zarathu.com/posts/2020-04-06-rdatamanagementtidyverse/), [data.table](https://blog.zarathu.com/posts/2022-02-11-datatable/) - 문서, 슬라이드 제작등 컨텐츠 만드는데 최적화. - [Rmarkdown](https://blog.zarathu.com/posts/2019-01-03-rmarkdown/): 글, 테이블, 그림, 참고문헌까지 R로만 논문 작성가능. - 공통데이터모델(OMOP-CDM) 분석언어 ] -- .large[ **AI 특화**는 Python 추천 - R로도 다 되긴하나(torch, keras), 구글링 Python 유리 - 신경망 외 일반적인 ML은 R로 충분히 좋다([caret](https://github.com/topepo/caret/) 추천) ] --- # Update: Python도 .large[ - [Quarto](https://quarto.org/): 파이썬, Juila도 Rmarkdown 처럼 - RStudio 사명 posit 으로 변경: 파이썬, Julia 지원 강화 ] --- # [tidyverse](https://blog.zarathu.com/posts/2020-04-06-rdatamanagementtidyverse/) .large[ R 기본문법 대체, 사실상 표준문법. ] <center> <a href="https://www.storybench.org/getting-started-with-tidyverse-in-r/"><img src="https://www.storybench.org/wp-content/uploads/2017/05/tidyverse.png" width=100%></a> </center> <center> <a href="http://ds4ps.org/dp4ss-textbook/p-072-data-recipes.html"><img src="http://ds4ps.org/dp4ss-textbook/figures/chaining_data_verbs.png" width=70%></a> </center> --- # [data.table](https://blog.zarathu.com/posts/2022-02-11-datatable/) .large[ 가장 빠른 스피드, 빅데이터용 필수패키지 - 심평원/공단분석 필수. - 파일 가장 빨리 읽고 쓰는 [fst](https://www.fstpackage.org/)와 찰떡궁합 - 멀티코어 지원 ] <center> <a href="https://github.com/fastverse/fastverse"><img src="https://pbs.twimg.com/media/E8mHra4VgA0vnY_.jpg" width=100%></a> </center> --- # [R plot](https://blog.zarathu.com/posts/2022-03-25-graph/) .large[ [ggplot2](https://ggplot2.tidyverse.org/) > Base: 사실상 표준 - [ggpubr](https://partrita.github.io/posts/ggpubr/) : 연구용 그림 + p-value 최적화 - [highcharter](https://jkunst.com/highcharter/): 반응형 그림, 상업용은 유료 - [jskm](https://github.com/jinseob2kim/jskm): 자체 개발 kaplan-meier 패키지 ] <center> <a href="https://github.com/jinseob2kim/jskm"><img src="https://github.com/jinseob2kim/jskm/raw/master/man/figures/README-unnamed-chunk-5-3.png" width=50%></a> </center> --- # PPT export: [officer](https://davidgohel.github.io/officer/) .large[ 그림 직접수정위해 반드시 PPT with 벡터그래픽 필요 ] ```r library(rvg);library(officer) editable_graph <- dml(ggobj = gg_plot) doc <- read_pptx() doc <- add_slide(doc) doc <- ph_with(x = doc, editable_graph, location = ph_location_type(type = "body") ) print(doc, target = "reports/rvg.pptx") ``` <center> <a href="https://ardata-fr.github.io/officeverse/static/reports/rvg.pptx"><img src="https://ardata-fr.github.io/officeverse/static/reports/rvg.png" width=40%></a> </center> --- # 공단/심평원분석 전략 .large[ 공단/심평원은 SAS 파일, 그러나 R로 대부분 가능 - [haven](https://haven.tidyverse.org) 패키지로 sas7bdat 읽기, but 느림 - csv 또는 fst로 변환하여 R로 빨리 읽기 - SAS는 R서버 RAM보다 큰 데이터 다룰 때만: 크게크게 쳐냄 - 반출 테이블: xlsx 하나에 탭들로 저장, [openxlsx](https://github.com/ycphs/openxlsx) 패키지 이용 - 반출 그림: pptx 하나에 여러 슬라이드로 저장. [officer](https://davidgohel.github.io/officer/) ] --- # [openxlsx](https://github.com/ycphs/openxlsx) ```r # create workbook wb <- createWorkbook() addWorksheet(wb = wb, sheetName = "htwt") writeData(wb = wb, sheet = "htwt", x = htwt) addWorksheet(wb = wb, sheetName = "smk") writeData(wb = wb, sheet = "smk", x = smk, rowNames=T) saveWorkbook(wb, "tables.xlsx", overwrite = T) ``` .large[ [반출 파일 실습](https://github.com/jinseob2kim/R-skku-biohrs/blob/main/lecture/dataexport.pptx?raw=true) ] --- # CDM(OMOP) with R .large[ CDM 분석 모든과정은 [R패키지](https://github.com/OHDSI)로 구현됨. ] <center> <img src="https://www.ohdsi.org/wp-content/uploads/2020/06/methodsLibrary.png" width=70%></a> </center> --- # 분석코드, 결과도 R패키지 .large[ 연구설계, 분석방법이 포함된 자체 R패키지가 코드공유 표준. - R패키지를 여러 기관에서 실행 ] https://github.com/zarathucorp/RanitidineCancerRisk -- .large[ 분석결과는 R Shiny로 - 실행결과를 R기반 웹애플리케이션으로 표현 - 분석코드에 웹코드도 포함되어있음. ] --- # 통계 .large[ 데이터 형태에 따라 - 일반, 반복측정, 표본추출, Propensity/IPTW 생존분석: Time-dependent analysis, landmark analysis 메타분석: [meta](https://bookdown.org/MathiasHarrer/Doing_Meta_Analysis_in_R/), [자체 개발앱](https://openstat.ai/app/meta-analysis/) 시계열분석: [fpp3 교과서](https://otexts.com/fpp3/)- ARIMA, [prophet](https://facebook.github.io/prophet/) ML general: [caret](https://topepo.github.io/caret/index.html) Non-linear(GAM) model: [mgcv](https://www.maths.ed.ac.uk/~swood34/mgcv/) ] --- # 분석(X), 결과테이블(O) ```r tb <- jstable::CreateTableOneJS(c("sex", "age", "status", "time"), strata = "rx", data = colon, nonnormal = "time") kable(tb$table[, 1:6]) ``` | |level |Obs |Lev |Lev+5FU |p |test | |:-------------------|:-----|:-------------------------|:-------------------------|:-------------------------|:------|:-------| |n | |630 |620 |608 | | | |sex (%) |0 |298 (47.3) |266 (42.9) |326 (53.6) |0.001 | | | |1 |332 (52.7) |354 (57.1) |282 (46.4) | | | |age | |59.45 ± 11.96 |60.11 ± 11.64 |59.70 ± 12.25 |0.608 | | |status (%) |0 |285 (45.2) |287 (46.3) |366 (60.2) |<0.001 | | | |1 |345 (54.8) |333 (53.7) |242 (39.8) | | | |time (median [IQR]) | |1506.00 [496.75, 2231.00] |1562.50 [498.75, 2332.00] |2066.00 [700.75, 2450.00] |<0.001 |nonnorm | --- # 표본추출(국건영) ```r nhanesSvy <- svydesign(ids = ~ SDMVPSU, strata = ~ SDMVSTRA, weights = ~ WTMEC2YR, nest = T, data = nhanes) tb <- jstable::svyCreateTableOneJS(c("HI_CHOL", "agecat"), strata = "RIAGENDR", data = nhanesSvy) kable(tb$table[, 1:5]) ``` | |level |1 |2 |p |test | |:-----------|:--------|:------------------|:------------------|:-----|:----| |n | |134944553.9 |141591892.0 | | | |HI_CHOL (%) |0 |112307738.4 (89.9) |114402926.5 (87.7) |0.010 | | | |1 |12579208.9 (10.1) |16056036.4 (12.3) | | | |agecat (%) |(0,19] |29299546.1 (21.7) |28150760.5 (19.9) |0.001 | | | |(19,39] |40497613.1 (30.0) |40640361.5 (28.7) | | | | |(39,59] |41053579.4 (30.4) |42817044.0 (30.2) | | | | |(59,Inf] |24093815.3 (17.9) |29983725.9 (21.2) | | | --- # 회귀분석류 table 만들기 ```r glm_gaussian <- glm(mpg~cyl + disp, data = mtcars) jstable::glmshow.display(glm_gaussian, decimal = 2)$table %>% kable ``` | |crude coeff.(95%CI) |crude P value |adj. coeff.(95%CI) |adj. P value | |:----|:-------------------|:-------------|:-------------------|:------------| |cyl |-2.88 (-3.51,-2.24) |< 0.001 |-1.59 (-2.98,-0.19) |0.034 | |disp |-0.04 (-0.05,-0.03) |< 0.001 |-0.02 (-0.04,0) |0.054 | ```r glm_binomial <- glm(vs~cyl + disp, data = mtcars, family = binomial) jstable::glmshow.display(glm_binomial, decimal = 2)$table %>% kable ``` | |crude OR.(95%CI) |crude P value |adj. OR.(95%CI) |adj. P value | |:----|:----------------|:-------------|:----------------|:------------| |cyl |0.2 (0.08,0.56) |0.002 |0.15 (0.02,1.02) |0.053 | |disp |0.98 (0.97,0.99) |0.002 |1 (0.98,1.03) |0.715 | [더 살펴보기](https://github.com/jinseob2kim/jstable) --- # Propensity score .large[ 그룹간의 baseline 맞춰주는 방법 - **Matching**: 적당한 사람들을 뽑음, N loss - **IPTW(Inverse probability treatment weighting)**: 사람마다 가중치를 다르게 줌, N loss 無 - [MatchIt](https://cran.r-project.org/web/packages/MatchIt/vignettes/MatchIt.html): 2그룹 PS matching, IPTW - [twang](https://cran.r-project.org/web/packages/twang/index.html) : 3그룹 이상 IPTW ] --- # [생존분석 심화](https://blog.zarathu.com/posts/2020-10-29-survivalpractice/) **Time-dependent coefficients** - 비례위험가정 깨질 때, Landmark analysis **Time-dependent covariate** - 관찰 시작 후 covariate 상태가 바뀔때 (ex: 약물복용) 예: 피부질환 진단 후(Indexdate) 약물치료 여부에 따른 Outcome - **Indexdate ~ 약물치료일, 약물치료일 ~ F/U 마지막날** 한 사람의 데이터를 2개로 나눔. Competing risk analysis: 최신 `survival` 패키지 기본옵션. - 심혈관질환 발생할 사람이었는데 죽어서 못본다? - 심혈관질환: outcome, 사망: competing risk --- # Landmark analysis ```r fit <- survfit(Surv(time,status)~rx, data=colon) jskm::jskm(fit, mark = F, surv.scale = "percent", pval =T, table = T, cut.landmark = 500, showpercent = T) ``` <img src="index_files/figure-html/unnamed-chunk-6-1.png" width="50%" style="display: block; margin: auto;" /> --- # Meta analysis .large[ [meta](https://github.com/guido-s/meta/) 패키지가 표준 - Forest/Drapery plot, Sub-group analysis - Random/Fixed effect - Publication bias: Funnel plot/Trim & fill ] [자체 개발앱](https://openstat.ai/app/meta-analysis/) --- # 시계열 .large[ [fpp3](https://otexts.com/fpp3/) 교과서 추천 - [tsibble](https://tsibble.tidyverts.org/): 시계열 데이터전처리 - [fable](https://fable.tidyverts.org/): ARIMA 계수 자동추정, 간단한 시계열 인공신경망 - [prophet](https://facebook.github.io/prophet/): Meta 가 개발한 Additive model 기반 패키지 ] <center> <a href="https://www.youtube.com/watch?v=6v3_AsbhqrE"><img src="https://i.ytimg.com/vi/6v3_AsbhqrE/maxresdefault.jpg" width=40%></a> </center> --- # ETC .large[ Non-linear(GAM) model - [mgcv](https://www.maths.ed.ac.uk/~swood34/mgcv/) 패키지가 표준 ML general - [caret](https://topepo.github.io/caret/index.html) ] --- # [Github](https://github.com/) .large[ 코딩하는 사람은 무조건 써야함. R보다 더 중요 - 코드 관리, 모든 중간기록 보관 - 오픈소스 SW(예: R패키지) 는 대부분 Github 프로젝트 - 포트폴리오 관리: 사실상의 이력서 - 간단한 홈페이지 운영 가능: 본 슬라이드도 Github 호스팅 ] --- class: center, middle # 서비스 --- # [R](https://www.r-project.org/)의 확장 .large[ [R](https://www.r-project.org/)은 이제 단순한 통계프로그램이 아님. * [R Markdown](https://rmarkdown.rstudio.com/): 논문과 발표자료를 [R](https://www.r-project.org/) 에서 직접 만듦. [과거 정리 내용](https://blog.zarathu.com/posts/2019-01-03-rmarkdown/) ] -- .large[ * [blogdown](https://github.com/rstudio/blogdown): 홈페이지도 만들 수 있음. 당사 홈페이지도 이것과 [distill](https://rstudio.github.io/distill/) 로 제작. ] -- .large[ * [Shiny](https://shiny.rstudio.com/), [Shiny Server](https://www.rstudio.com/products/shiny/shiny-server/): 서버 구축하고 웹기반 앱을 만들 수 있음. 간단한 Shiny 앱들은 Shinyapps.io 에서 무료호스팅. ] -- 법인 설립 후 모든 작업에 [R](https://www.r-project.org/)을 이용함. --- # 논문 .large[ [R](https://www.r-project.org/)에서 글, 그림, 테이블, 참고문헌까지 직접 작성. * [rticles](https://github.com/rstudio/rticles): 대부분 학술지의 템플릿을 포함. <img src="https://bookdown.org/yihui/rmarkdown/images/rticles-templates.png" width="50%" style="display: block; margin: auto;" /> ] [준비하던 박사논문](https://blog.zarathu.com/posts/2018-11-08-mdlm/) --- # 발표 슬라이드 .large[ 논문쓸 때 이용했던 R 코드 활용, 빠르게 발표 슬라이드 제작 가능. * [기본 템플릿](https://rmarkdown.rstudio.com/lesson-11.html), [xaringan](https://github.com/yihui/xaringan) 패키지: 이 슬라이드도 [xaringan](https://github.com/yihui/xaringan)으로 제작. <img src="https://user-images.githubusercontent.com/163582/53144527-35f7a500-3562-11e9-862e-892d3fd7036d.gif" width="70%" style="display: block; margin: auto;" /> 포스터: [posterdown](https://brentthorne.github.io/posterdown_html_showcase/), [pagedown](https://pagedown.rbind.io/poster-relaxed) ] --- # 발표 슬라이드: revealjs .large[ 새로만드는 슬라이드는 [revealjs](https://quarto.org/docs/presentations/revealjs/)로 작업 <iframe src="https://jinseob2kim.github.io/lecture-general/APPFGH2022" width="100%" height="300px"></iframe> ] --- # 홈페이지 .large[ [blogdown](https://github.com/rstudio/blogdown), [distill](https://rstudio.github.io/distill/): [깃허브](https://pages.github.com/) 통해 무료 웹호스팅 가능. <img src="https://www.storybench.org/wp-content/uploads/2019/05/blogdown-new-site-e1557149319624-730x457.png" width="80%" style="display: block; margin: auto;" /> [홈페이지](https://github.com/zarathucorp/zarathu), [블로그](https://github.com/zarathucorp/blog) ] --- # 웹 앱 .large[ [Shiny](https://shiny.rstudio.com/): [R](https://www.r-project.org/) 코드만으로 웹앱 제작 ] <img src="https://shiny.rstudio.com/images/debugging/kmeans-showcase.gif" style="display: block; margin: auto;" /> --- class: center, middle # Shiny 소개 --- ### [Shiny](https://shiny.rstudio.com/) is an R package that makes it easy to **build web applications with R** <img src="https://github.com/jinseob2kim/shiny-workshop-odsc2019/raw/master/docs/rs.png" style="display: block; margin: auto;" /> --- # 웹 개발 지식 없어도 됨 <img src="https://github.com/jinseob2kim/shiny-workshop-odsc2019/raw/master/docs/css.png" style="display: block; margin: auto;" /> --- # Shiny app <img src="https://github.com/jinseob2kim/shiny-workshop-odsc2019/raw/master/docs/ui.png" style="display: block; margin: auto;" /> --- # UI & SERVER <img src="https://github.com/jinseob2kim/shiny-workshop-odsc2019/raw/master/docs/ui2.png" style="display: block; margin: auto;" /> --- # Make basic App * `app.R` 파일 만들고(**이름 바뀌면 안됨**) * `ui` 와 `server` 코드 작성 후 `shinyApp` 으로 마무리. **Run App** 눌러 실행. - `ui.R`, `server.R` 따로 작성할 수도 있음. 개인 취향 <img src="https://github.com/jinseob2kim/shiny-workshop-odsc2019/raw/master/docs/basic.png" style="display: block; margin: auto;" /> --- # 공부모임: Shiny 밋업 .large[ https://github.com/shinykorea/Meetup - 월 1회 공부내용 공유. 36회 진행 - 의료/유전학/축산/반도체/게임/IPTV/회계 등 다양한 분야 사람들이 모임. - **경기도 코로나 대시보드 공동작업** - 21년 공개SW기반 **커뮤니티** 지원사업, **韓中日 공개SW** 국제협력 강화 TASK 선정 ] --- class: center, middle # 서버 --- # 분석환경 .large[ 빅데이터분석은 대부분 리눅스 서버에서 - 공단/심평원 R server 서버 초기세팅(RStudio, 패키지, Shiny 설치) 위한 리눅스 지식(ubuntu or fedora) ] --- # 워크샵 계획 <center> <a href="https://education.zarathu.com/"><img src="r4cr.png" width=100%></a> </center> --- # 서버 바뀌면? .large[ 서버 바뀔때 마다 새로 세팅? - R 패키지 설치에만 1시간 이상 걸림(리눅스는 오래걸림) 아예 초기세팅 완료된 가상머신을 만들어놓자. 그러나 Virtual Machine(VM) 은 너무 느림 - [Docker](https://www.docker.com/)는 리눅스기반 컨테이너 서비스. - [rocker project](https://www.rocker-project.org/): R 관련 Docker image 모음 - RStudio, 패키지, Shiny server 모두 설치된 [rshiny image](https://github.com/jinseob2kim/docker-rshiny) 자체 개발 ] --- # Docker .large[ 마이크로서비스 아키텍처란? ] <img src="https://blog.philipphauer.de/blog/2015/0411-microservices-nutshell-pros-cons/Monolith-vs-Microservices.png" width="40%" style="display: block; margin: auto;" /> <div class="figure" style="text-align: center"> <img src="https://blog.philipphauer.de/blog/2015/0411-microservices-nutshell-pros-cons/Scaling-Microservices.png" alt="https://blog.philipphauer.de/microservices-nutshell-pros-cons/" width="60%" /> <p class="caption">https://blog.philipphauer.de/microservices-nutshell-pros-cons/</p> </div> --- # 여행용 파우치 <div class="figure" style="text-align: center"> <img src="https://m.onpan.co.kr/web/product/big/202104/ed2cf34728610b0aa0ca04301ff9051a.jpg" alt="https://funshop.akamaized.net/products/0000045775/HF-INLUGGAGE-POUCH-LINGERIE-%EC%83%81%EC%84%B8%ED%8E%98%EC%9D%B4%EC%A7%80_01.jpg" width="60%" /> <p class="caption">https://funshop.akamaized.net/products/0000045775/HF-INLUGGAGE-POUCH-LINGERIE-%EC%83%81%EC%84%B8%ED%8E%98%EC%9D%B4%EC%A7%80_01.jpg</p> </div> --- # 여행용 파우치 장단점 장점 1. **깔끔하다.** 2. 치우기 쉽다. 3. 다른 가방으로 옮기기 쉽다. 4. 가방 종류에 구애받지 않는다. 단점 1. 실제 쓸 수 있는 공간이 줄어든다. 2. 분리해서 넣기 귀찮다. 3. 물건 찾을 때 지퍼를 한번 더 열어야 된다. --- # Microservice 장단점 장점 1. **깔끔하다.** 2. 삭제가 쉽다. 3. 다른 컴퓨터에 재설치 쉽다. 4. 컴퓨터/서버 종류에 구애받지 않는다. 단점 1. 실제 쓸 수 있는 용량이 줄어든다. 2. 서비스마다 모듈 만들기 귀찮다. 3. 성능저하 우려 **가상머신(Virtual machine)** 활용이 대표적. --- # [Docker](https://www.docker.com/what-docker) - 빠르고 용량이 적은 가상머신? - [Docker hub](https://hub.docker.com/)을 통해 [github](https://github.com/)처럼 이용가능. + [github](https://github.com/)과 연계 가능 + [github](https://github.com/)에 이미지 제작 코드 저장하면 [Docker hub](https://hub.docker.com/)에 실제 이미지가 저장 <div class="figure" style="text-align: center"> <img src="https://journals.plos.org/plosone/article/figure/image?size=large&id=10.1371/journal.pone.0152686.g002" alt="https://doi.org/10.1371/journal.pone.0152686" width="60%" /> <p class="caption">https://doi.org/10.1371/journal.pone.0152686</p> </div> --- # 클라우드 .large[ 물리서버 필요없음, 쓴만큼 요금부과 - AWS, Azure, 네이버, [vultr](https://www.vultr.com/)(한국 region 있으면서 가장저렴) OMOM-CDM 실습환경이 [AWS](https://github.com/OHDSI/OHDSIonAWS)로 제공됨 [openstat.ai](https://openstat.ai) 도 클라우드에서 운영 ] <center> <a href="https://github.com/OHDSI/OHDSIonAWS"><img src="https://raw.githubusercontent.com/OHDSI/OHDSIonAWS/master/images/ohdsi_architecture_block_diagram.png" width=55%></a> </center> --- # 발표: 개발환경구축 <center> <a href="https://blog.zarathu.com/posts/2018-11-08-ruck2018/"><img src="https://raw.githubusercontent.com/jinseob2kim/lecture-general/master/docs/snu-premed/RUCK2018.jpg" width=80%></a> </center> --- # Executive summary .large[ 분석: **의학 + 연구는 R**추천 - 데이터 전처리는 **[data.table](https://blog.zarathu.com/posts/2022-02-11-datatable/)** 과 `%>%`. - R로 ppt/xlsx 만들기(분석결과 반출) - 일반, 반복측정, 표본추출, Propensity, meta, 시계열 - 코드관리는 Github 서비스: 리포트(Rmarkdown), 웹 애플리케이션(Shiny) 서버: 분석환경 or 웹 서비스 - 리눅스, 클라우드, Docker ] --- # 철강왕 박태준 우향우정신 .large[ > 선조의 피값(대일 청구권 자금)으로 짓는 포항제철, 실패하면 우향우해서 영일만에 빠져 죽자 ] <center> <img src="https://pds.joongang.co.kr/news/component/htmlphoto_mmdata/201511/29/htm_2015112912557808759.jpg" width=40%></a> </center> --- # 醫俠(의협: 의학 + 의로울 협) 정신 .large[ > 환자 희생으로 만들어진 데이터, 실패하면 한강에 빠져 죽자 - 최선의 결과를 내야하는 의료진, 최선의 결과를 원하는 환자 - But, 최악과 차악뿐인 선택지 - 선택과 사투과정이 담긴 데이터 - 이 데이터를 헛되게 쓰는건, 환자의 희생과 의료진의 노력을 헛되게 만드는 것. ] --- class: center, middle # END